News
Artificial intelligence and energy usage: crisis or catalyst?
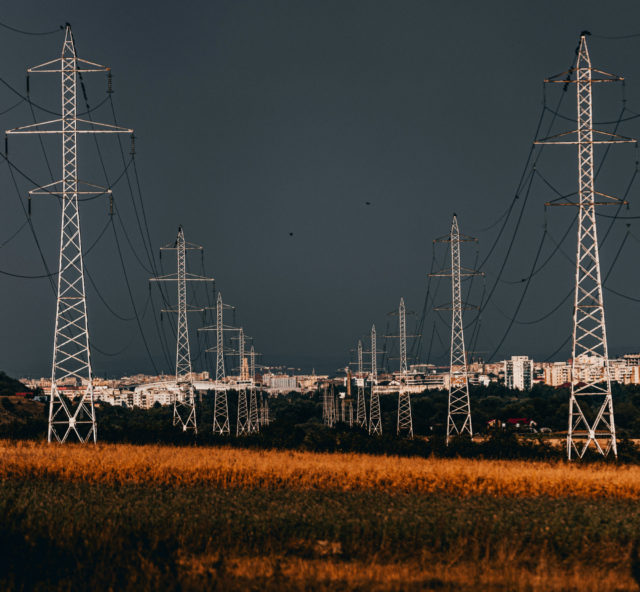
DeepSeek, a hedge fund-backed Chinese artificial intelligence company, seemed to emerge as an unlikely ally for environmental activists when they released their most recent large language model (LLM) in January 2025. The model promised GPT-level accuracy with fewer chips, less energy, and a smaller budget.
Excitement abounded as people regaled this innovation as the end of AI’s energy woes. But how was AI causing increased energy usage in the first place? Is DeepSeek the silver bullet we’ve been waiting for?
This blog post will take you through a brief history, covering age-old fears about new technologies, the innovation currently changing AI’s relationship with energy, and the future for AI as a tool in the green technology shed. By acknowledging AI’s environmental challenges while framing them within the net-zero transition and alongside other energy-intensive technologies, this article aims to leave you more informed—and perhaps a bit more hopeful.
AI’s environmental footprint in the spotlight
Since OpenAI went public with the GPT series in late 2022, Artificial Intelligence, or more specifically and accurately, generative AI has increased demand on data centres around the world. It was predicted that Americans would see their energy costs go up by as much as 70%, and that energy demand would increase 16% in 5 years. Additionally, there have been concerns about water usage. Shaolei Ren, an associate professor of electrical and computer engineering at UC Riverside, estimates a session (roughly 10 to 50 responses) with GPT-3 could consume half a litre of freshwater. Tech company leaders like Microsoft President Brad Smith stoked the fear, saying, “In 2020, we unveiled what we called our carbon moonshot. That was before the explosion of artificial intelligence.” Headlines like *AI’s Energy Demands Are Out of Control. Welcome to the Internet’s Hyper-Consumption Era* belied a feeling of inherent doom about the climate in an age of AI.
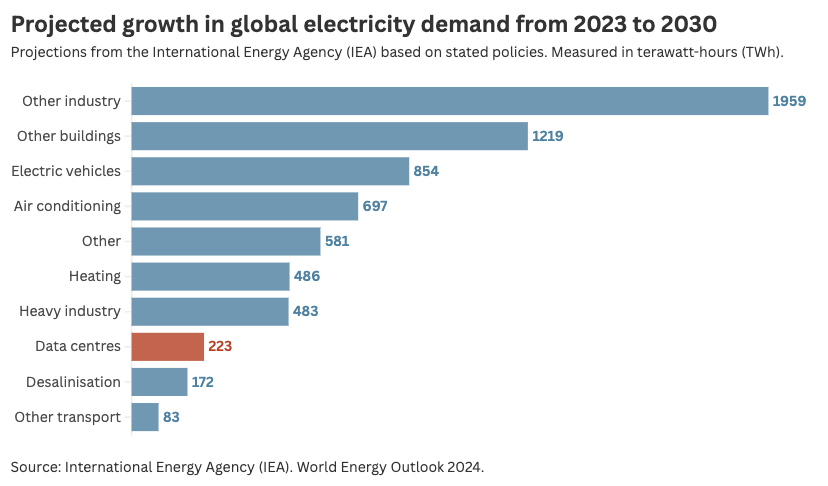
What is generative AI?
“Generative AI” is the more accurate term for what most people have come to know as simply “AI.”
It includes chatbots like ChatGPT, image generation models like DALL-E, and Google’s ‘AI Overview.’ Essentially, the modifier ‘generative’ can be applied to any model that produces new content—whether text, images, or code—rather than simply analysing or classifying existing data.
You might know it for producing images like this:
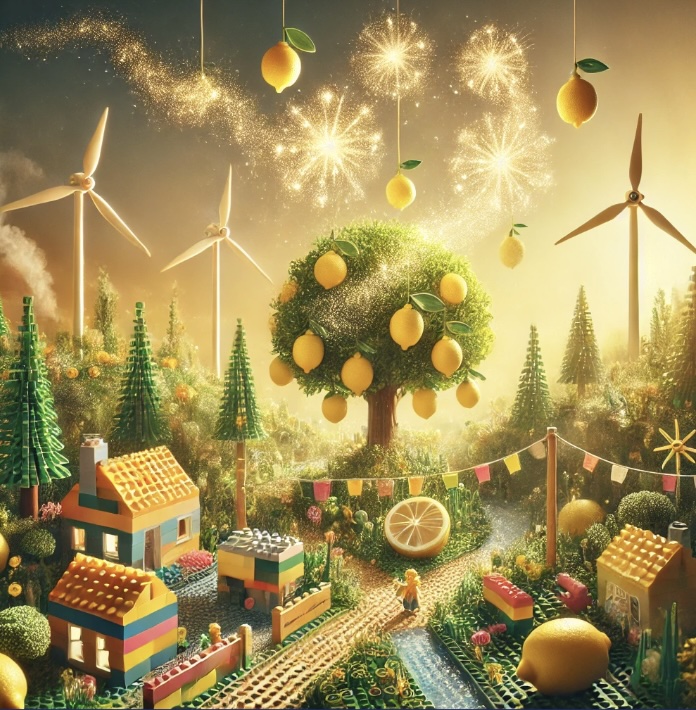
or poems like this:
Fairy dust glimmers,
Softly stitching skies with gold.
Lemons bloom like suns,
Life’s zest in their tangy hold.
Legos stack with care,
Bricks of hope and dreams align.
Harmony is built,
A world of balance, divine.
Of course, generative AI’s use cases can get much more nefarious than coming up with poems about magical lemon-lego worlds. And its integration into daily life can be subtle. As I type this blog on Notion, a popular productivity and note-taking app, it helpfully encourages me to “Improve writing” with the press of a button, complete with a magic wand icon. But these technologies are not magic, they are the product of vast datasets, complex algorithms, and immense computational power. Their complexity is directly tied to their energy usage and generative AI is the most energy intensive type of AI.
As such, generative AI models are on center stage in this blog but it is always worth remembering they are not the only AI tool. Other tools, like those used in environmental monitoring, autonomous driving, genetics research, medical imaging, and operational efficiency focus on analyzing patterns. Typically accessed through an API or user-interface, these tools may not be immediately obvious as AI, as they lack the large language model component we have come to associate with AI in the public imagination.
If you can’t tell whether or not you are using generative AI, a good rule-of-thumb is to consider whether or not you feel like you are ‘speaking’ to the model. If you are, it is likely generative.
The tech party that’s driving up energy demand
However, this narrative ignores the fact that AI is just one attendee at a party of technologies requiring more energy than ever before. Among them are big names in the environmental world like electric vehicles, desalination and water purification techniques, and electric heating and cooling systems. In fact, one team of researchers forecasted electricity demand for a business-as-usual scenario versus a scenario that sees industry align with net-zero targets. Electricity was projected at a 24% increase in the latter over the former.
Other technologies like cryptocurrencies, 5G telecommunication networks, and space exploration also want to party like it’s 1999, increasing technological energy consumption and making people fear the end of the world along the way. As Casey Crownhart of the MIT Technology Review puts it (in an article provocatively titled *AI is an energy hog. This is what it means for climate change.)*, “AI is probably going to be a small piece of a much bigger story. Ultimately, rising electricity demand from AI is in some ways no different from rising demand from EVs, heat pumps, or factory growth. It’s really how we meet that demand that matters.”
“AI is probably going to be a small piece of a much bigger story. Ultimately, rising electricity demand from AI is in some ways no different from rising demand from EVs, heat pumps, or factory growth. It’s really how we meet that demand that matters.”
Casey Crownhart, MIT Technology Review
Efforts should be made to ensure computing technology requires less energy. But, more importantly, efforts should be redoubled to decarbonise the grid. Grid decarbonisation is crucial as new technologies, and electricity-intensive ones, prove part of the sustainability solution. For example, direct air capture (DAC) technology, which uses chemical processes to remove CO2 from the atmosphere, consumes up to 2000 kWh per ton of CO2 captured. For comparison, a typical household consumes approximately 10000 kWh in a year. But DAC is also essential for meeting net zero targets, underpinning scenario models attempting to curtail warming below 1.5˚C above pre-industrial levels. Climeworks, a leader in the industry, power their CO2-removal technology using geothermal energy. AI companies and data centers should follow suit. The carbon footprint of AI depends largely on when and where it runs, with fossil-heavy grids vastly increasing emissions.
The internet was supposed to crash the energy grid too
The similarities with the turn of the century also echo media fears about the energy demands of new technology. As pointed out by Michael Thomas in his piece on AI and the environment, this is not the first time the world has worried about the energy implications of a new technology. 1999 saw headlines like Dig more coal — the PCs are coming, an article that would go on to predict the internet would soon use up half of the American electrical grid. In 2024, data centres in the U.S. used closer to 4%.
It begs the question—how did these journalists (and they weren’t the only ones) get it so wrong? Well, they predicted a linear trajectory; an increased demand commensurate with the growth of the product in the same form in which it originally came to market. They did not account for the innovation that typifies the tech industry. Doing things with less energy doesn’t just entail environmental stewardship, it is also friendly to corporate bottom lines. That’s because, as anyone who has been scolded by a parent or partner to turn the lights off before leaving the house can tell you, electricity costs money. And working with fewer resources was exactly DeepSeek’s mission. The potential energy savings are just a happy side effect. But they mirror the happy side effects of innovations of the 00s—creating more energy-efficient semiconductor chips, techniques to require less water for cooling in data centres, and companies moving away from inefficient self-hosting.
When a new technology comes to market, it is impossible to say how energy consumption will change. That technology’s technical ecosystem will develop and reliance on legacy technology, built for other purposes, will wane. The technology underpinning AI is no different. Although they are far from being made obsolete in AI innovations at present, Graphic Processing Units (GPUs) are a good example. Today, GPUs are sought after and tightly controlled for their ability to power LLMs, but they were originally built for graphics, effects, and video games.
AI was always going to change form, slim down, and become more energy efficient because it’s economically sensible, and DeepSeek is the first piece of evidence that time and tide wait for no man.
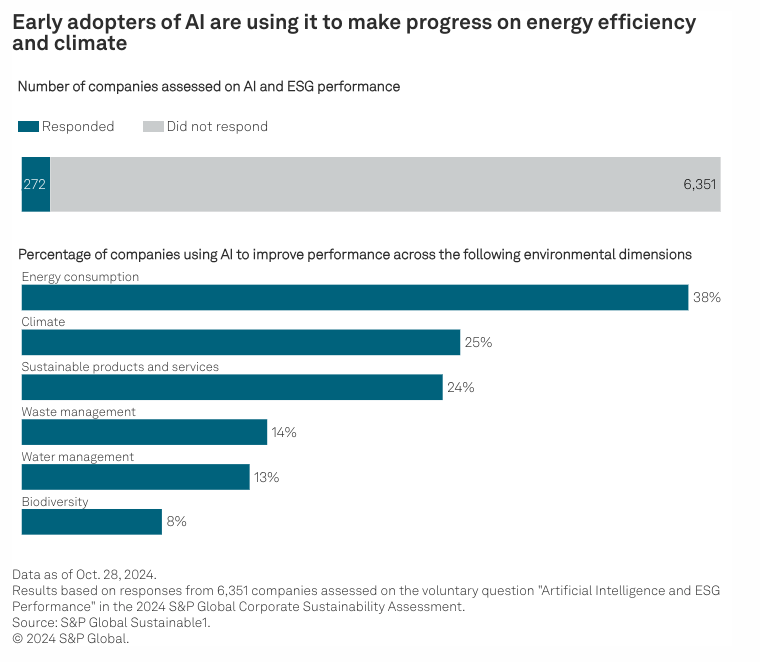
AI for a greener future
Counterintuitively to some, AI actually has the potential to aid sustainability ambitions. According to the 2024 S&P Global Corporate Sustainability Assessment, 38% of companies who responded to the survey are using AI to improve performance related to energy consumption. Responding companies are also using it for climate, sustainable products and services, waste management, water management, and biodiversity.
I was grateful to be surrounded by the innovative minds behind environmentally applied machine learning in my Master’s program. Peers made models to predict the movement of tropical cyclones, estimate and improve the energy efficiency of buildings, and optimise agricultural practices to enhance crop resilience. For my dissertation, I used machine learning to improve transparency in the voluntary carbon market by estimating forest-based carbon sequestration potential at the individual tree level.
Here at TCLP, we have used machine learning to identify climate-related clauses in existing contracts and to propose climate-related clauses to those who are not already using them (termed internally the ‘Clause Detector’ and ‘Clause Recommender’ respectively).
Many of these environmentally oriented applications are non-generative and therefore not particularly energy-intensive. To speak only for what we are doing at TCLP, it is non-generative, low-risk, and its energy usage is approximately akin to boiling an electric kettle for 11 seconds.
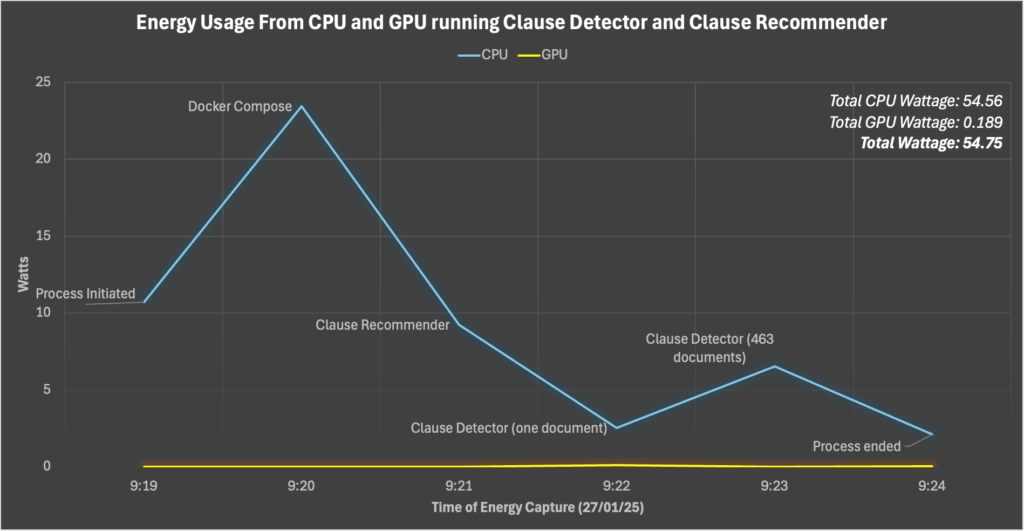
A Note on the Challenge of Calculating the Energy Usage of AI
Accurately accounting for the energy usage of AI, especially in the face of its estimated environmental impacts, has been a challenge tackled by multiple teams of researchers. The prevailing thought—it is not as easy as it may seem.
Imagine you have a laptop training a machine learning algorithm. Well, there could be emissions differences from:
- Hardware Configuration – CPU, GPU, memory, and cooling all affect power use, even between seemingly identical devices.
- Measurement Method – At-the-wall power meters, software tools, and proxies all yield different estimates and have pros and cons to their usage.
- Background Processes – Other apps and system tasks can skew energy measurements, including if a software-based measurement tool is being used. The measurement tool itself will use some energy.
- Grid Variability – The carbon footprint of AI depends on when and where it runs; fossil-heavy vs. renewable-powered grids make a huge difference.
These pressure points for variability are summarised from Jagannadharao et al. (2024) and do not completely cover the measurement issues the paper identifies. But they are a taste for the inexactitude plaguing energy usage tracking in computing generally and especially applied to AI.
With no universal standard for AI energy tracking, readers should be wary that widely cited figures and metaphors can be, at worst, misleading and, at best, uncertain.
There is also a difference in training AI versus deploying it for usage. The former is much more energy intensive but also done much less frequently.
The graph above, which demonstrates the approximate energy usage of the TCLP AI products at usage was calculated using real-time power monitoring with powermetrics on macOS, stored and analysed using InfluxDB via the InfluxDBClient Python library. The program was run on a MacBook Air with M2 chip. The script leverages subprocess to run power sampling at 300ms intervals, collecting both CPU and GPU power consumption. The specifics of power estimation (i.e. which program uses the most energy) is somewhat occluded by the use of a Docker container in launching the interface, as Docker integrates abstraction and shared layers.
This approach has undoubted limitations but is a starting point, proxy measurement of absolute energy (defined as a power estimate taking into account all power consumed, even background processes). As such, the above graph should mostly be referenced as an order of magnitude, rather than exact measurement of certain steps in the process. The total wattage used by the system when running all three programs, 54.56 Wh, is equivalent to that which a MacBook Air would use running any standard application.
Innovation in a changing world
Given my professional background and current academic interests, social gatherings often find me fielding AI opinions, ranging from garden-variety fear to grating explanations from people with only a passing acquaintance with the technology. Persistently, across demographics, there is a language issue. People are wary of this new technology because they don’t feel confident about what it is or how to explain it. This problem has only been exacerbated by marketing efforts that put a “powered by AI” label on everything from boilerplate non-intelligent algorithms to true large language models.
If we reduce AI to an abstract, catch-all term rather than understanding its nuances, we risk either overhyping its dangers or underestimating its potential to enhance climate action.
Misunderstanding AI’s capabilities and limitations can lead to misplaced fears, misguided regulations, or even the wrong investments in technology. The reality is that AI is neither a singular villain nor a silver bullet. Its environmental impact depends on how we wield it and how we innovate. If we reduce AI to an abstract, catch-all term rather than understanding its nuances, we risk either overhyping its dangers or underestimating its potential to enhance climate action. In fact, AI itself could be the key to a smarter, greener grid.
As I tell the innocent and ill-informed alike, the train has left the station. The sustainability community needs to decide whether we’re going to run to catch it.
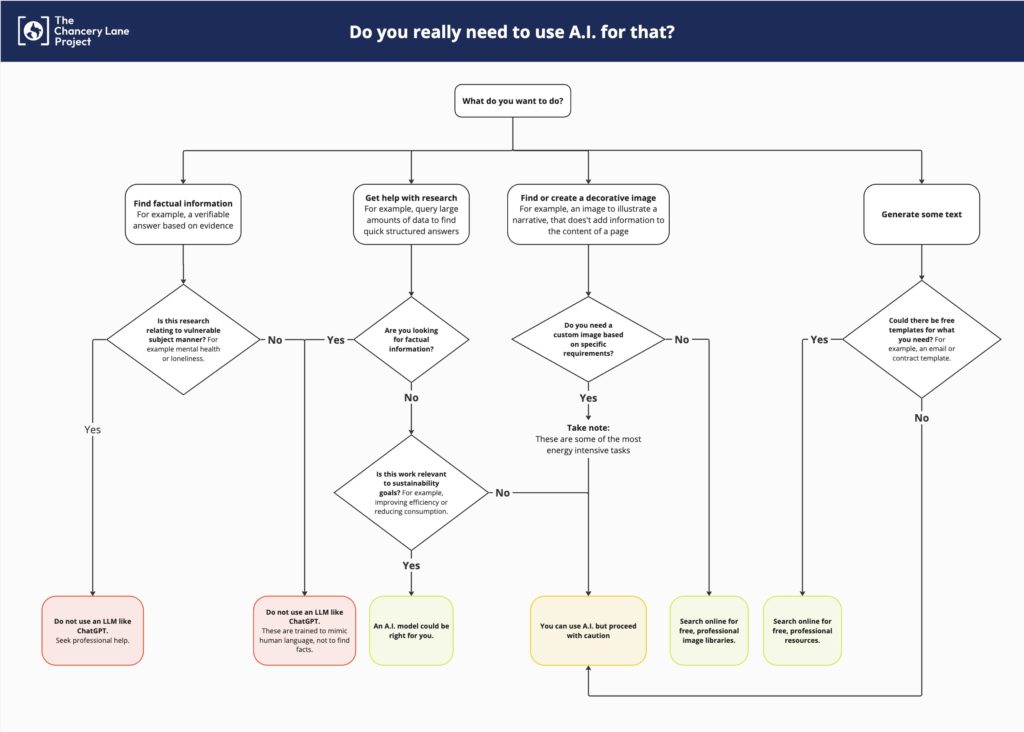
Georgia Ray began working with The Chancery Lane Project in autumn 2024 while on placement from Faculty. She has since joined the team as a Data Scientist while simultaneously pursing her PhD in environmental policy from Imperial College London, focusing on using machine learning to downscale deep decarbonisation pathways to sector-specific metrics. She received a Masters with Distinction, also from Imperial, in Environmental Data Science and Machine Learning and worked previously as a Research Associate for an American environmental non-profit, the Environmental Law Institute.